Data Warehousing on AWS
Data Warehousing on AWS introduces the concepts, strategies, and best practices for designing a cloud-based data warehousing solution using Amazon Redshift, AWS’ petabyte-scale data warehouse. This course shows you how to collect, store, and prepare data for your data warehouse using AWS services such as Amazon DynamoDB, Amazon EMR, Amazon Kinesis, and Amazon S3. Additionally, the course demonstrates how to use Amazon QuickSight to perform data analytics.
COD: AW-DWAWS
Categorie: AWS
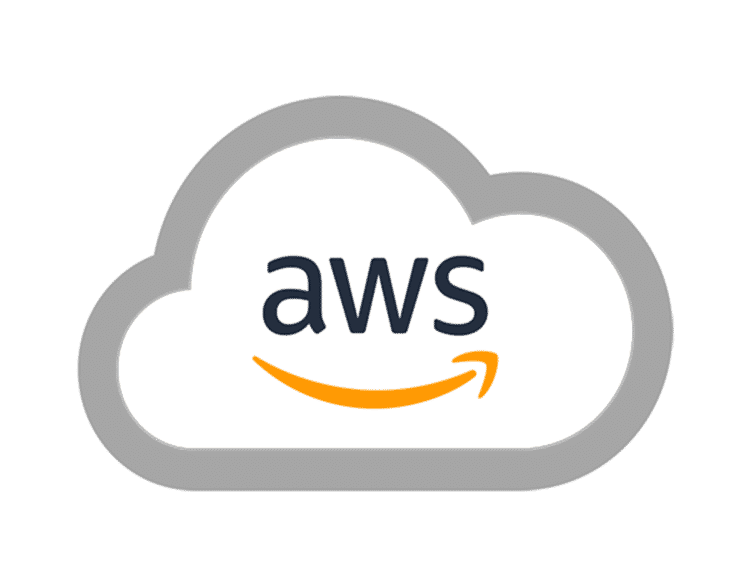
Who should participate
- Database architects
- Database administrators
- Database developers
- Data Analyst
Prerequisites
- Familiarity with relational databases and database design concepts
In this course you will learn to:
- Discuss the fundamental concepts of data warehousing and the intersection between data warehousing solutions and big data.
- Launch an Amazon Redshift cluster and use the components, features, and functionality to implement a cloud data warehouse.
- Use other AWS data and analytics services, such as Amazon DynamoDB, Amazon EMR, Amazon Kinesis, and Amazon S3, to contribute to your data warehousing solution.
- Design the data warehouse
- Identify performance issues, tune queries, and tune the database for better performance.
- Use Amazon Redshift Spectrum to analyze data directly from an Amazon S3 bucket.
- Use Amazon QuickSight to perform data visualization and analysis tasks on your data warehouse.
Day 1
Module 1: Introduction to Data Warehousing
- relational databases
- Data warehousing concepts
- The intersection of data warehousing and big data
- Overview of data management in AWS
- Hands-on Lab 1: Introduction to Amazon Redshift
Module 2: Introduction to Amazon Redshift
- Conceptual overview
- Real use cases
- Hands-on Lab 2: Launching an Amazon Redshift Cluster
Module 3: Starting Clusters
- Creating the cluster
- Connection to the cluster
- Access control
- Database security
- Upload data
- Hands-on Lab 3: Optimizing Database Schemas
Day 2
Module 4: Database schema design
- Patterns and data types
- Column compression
- Data distribution styles
- Data sorting methods
- Module 5: Identification of data sources
- Overview of data sources
- Amazon S3
- Amazon DynamoDB
- Amazon EMR
- Amazon Kinesis Data Firehose
- AWS Lambda database loader for Amazon Redshift
- Hands-on Lab 4: Loading Live Data into an Amazon Redshift Database
Module 6: Data Upload
- Data preparation
- Loading data with COPY
- Table maintenance
- Simultaneous write operations
- Loading troubleshooting
- Hands-on Lab 5: Loading Data with the COPY Command
Day 3
Module 7: Writing Queries and Optimizing Performance
- Amazon Redshift SQL
- User Defined Functions (UDF)
- Factors affecting query performance
- The EXPLAIN command and query plans
- Workload Management (WLM)
- Hands-on Lab 6: Configuring Workload Management
Modulo 8: Amazon Redshift Spectrum
- Amazon Redshift Spectrum
- Data configuration for Amazon Redshift Spectrum
- Query di Amazon Redshift Spectrum
- Hands-on Lab 7: Using Amazon Redshift Spectrum
Module 9: Cluster maintenance
- Audit logging
- Performance monitoring
- Events and notifications
Lab 8: Cluster Monitoring and Auditing
- Cluster scaling
- Cluster backup and recovery
- Labeling of resources, limits and constraints
- Hands-on Lab 9: Backing Up, Restoring, and Scaling Clusters
Module 10: Data Analysis and Visualization
- Power of views
- Creating dashboards
- Amazon QuickSight editions and features
Duration – 3 days
Delivery – in Classroom, On Site, Remote
PC and SW requirements:
- Internet connection
- Web browser, Google Chrome
- Zoom
Language
Instructor: English
Workshop: English
Slides: English